What is the obesity rate in Italy
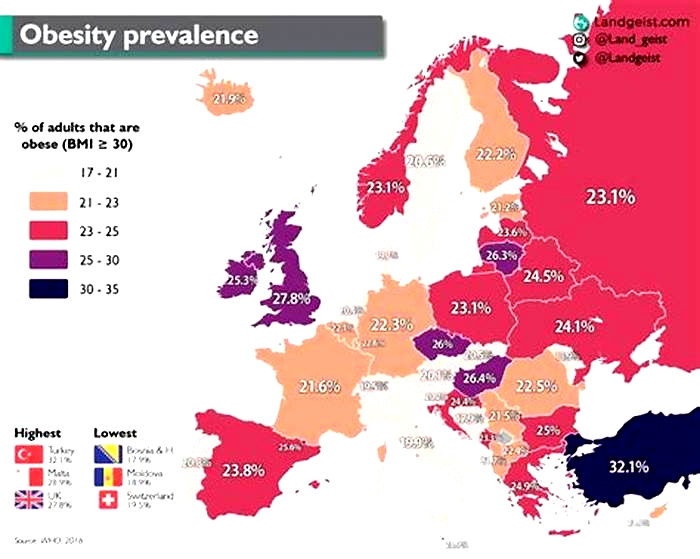
Italy
National Prevention Plan 2020-2025
The "National Prevention Plan 2020-2025", adopted with the State-Regions Agreement of 6 August 2020
Categories: | Evidence of Nutritional or Health Strategy/ Guidelines/Policy/Action plan |
Year(s): | 2020-2025 |
Target age group: | Adults and children |
Organisation: | Ministry of Health |
Findoutmore: | www.epicentro.iss.it |
Linkeddocument: | Download linked document |
Proposed alternative front of package labelling
New battery label based on 'whole food' rather than constitutents of food, has been approved for Italy by the EU. Currently being harmonised into the system with full integration by 2022
Categories: | Labelling Regulation/Guidelines |
Year(s): | 2020 (ongoing) |
Target age group: | Adults and children |
Findoutmore: | ec.europa.eu |
Annex III to Regulation (EC) No 1925/2006 of the European Parliament and of the Council as regards trans fat, other than trans fat naturally occurring in fat of animal origin
Measures to limit or virtually eliminate industrially-produced trans fatty acids in food intended for the final consumer and/or for supply to retail. Adopted by The European Commission from April 2019. (Available in multiple languages)
Categories: | Evidence of Marketing Guidelines/Policy |
Year(s): | 2019 (ongoing) |
Target age group: | Adults and children |
Organisation: | The European Commission |
Linkeddocument: | Download linked document |
References: | Information provided with kind permission of WHO Global database on the Implementation of Nutrition Action (GINA): https://extranet.who.int/nutrition/gina/en/node/36162 (last accessed 12.07.22) |
Physical activity guidelines
Guidelines on physical activity for different age groups and with reference to physiological and pathophysiological situations and specific subgroups of the population.
Categories: | Evidence of Physical Activity Guidelines/Policy |
Year(s): | 2019 (ongoing) |
Target age group: | Adults and children |
Organisation: | Ministry of Health |
Linkeddocument: | Download linked document |
Give me 5 Campaign
An educational campaign to prevent obesity in children, by teaching the importance of a healthy, balanced diet.
Guidelines for healthy eating
In 2018, the Food and Nutrition Research Centre released the revised version of 'Linee guida per una sana alimentazione', the Italian nutritional guidelines for healthy eating.
Categories: | Evidence of Nutritional or Health Strategy/ Guidelines/Policy/Action plan |
Year(s): | 2018 (ongoing) |
Organisation: | Food and Nutrition Research Centre |
Linkeddocument: | Download linked document |
Linee guida per una sana alimentazione 2018
More than ten years after the last revision, the 2018 revision of the Guidelines for healthy eating is published. It is the Italian reference document on healthy eating aimed at consumers. It collects and periodically updates a series of food recommendations and indications, elaborated by a specific scientific commission, made up of prestigious scholars from the world of nutrition and beyond
Categories: | Evidence of Nutritional or Health Strategy/ Guidelines/Policy/Action plan |
Year(s): | 2018 (ongoing) |
Target age group: | Adults and children |
Organisation: | Ministry of Health |
Findoutmore: | www.crea.gov.it |
School Fruit, Vegetables and Milk Scheme
"Applicable since 1 August 2017, the EU school fruit, vegetables and milk scheme combines two previous schemes (the school fruit and vegetables scheme and the school milk scheme) under a single legal framework for more efficiency and an enhanced focus on health and educational. The scheme supports the distribution of products, educational measures and information measures.The scheme supports the distribution of fruit, vegetables, milk and certain milk products to schoolchildren, from nursery to secondary school. EU countries approve a list of products (in collaboration with their health and nutrition authorities) which will help achieve the schemes objective of helping children to follow a healthy diet.Priority is for fresh fruit and vegetables and for plain milk. In order to support a varied diet and/or specific nutritional needs, EU countries may also make processed fruit and vegetables such as juices and soups and certain milk products such as yoghurt and cheese, available. Additionally, under stricter conditions, milk-based drinks may be included." - EU Commission
Shared objectives for the improvement of nutritional characteristics of food products with particular attention to the population of children (3-12 years)
In 2004, the Self-Regulatory Code of Conduct on Commercial Communication was expanded to protect children's nutrition. The regulations apply for children aged 312 years old and indicate that marketing aimed at children should not encourage or imply unhealthy dietary behaviours through product design and packaging. The Ministry of Health later developed guidelines to inform the implementation of the voluntary marketing code.
EU Regulation 1169/2011
Passed in 2011, EU Regulation 1169/2011 on the Provision of Food Information to Consumers requires a list of the nutrient content of most pre-packaged food to be provided on the back of the pack from October 2011.
Categories: | Labelling Regulation/Guidelines |
Year(s): | 2016 (ongoing) |
Target age group: | Adults and children |
Organisation: | European Parliament and the Council of the European Union |
Findoutmore: | eur-lex.europa.eu |
References: | Information provided with kind permission of WHO Global database on the Implementation of Nutrition Action (GINA): https://extranet.who.int/nutrition/gina/en/node/22917 (last accessed 28.06.22) |
GNPR 2016-2017: Infant and young child nutrition - Breastfeeding promotion and/or counselling
This programme was reported by countries for the 2nd WHO Global Nutrition Policy Review 2016-2017 module on actionsrelated to infant and young child nutrition. Information provided by WHO GINA Programme.
Categories: | Evidence of Breastfeeding promotion or related activity |
Year(s): | 2016 (ongoing) |
Target age group: | Children |
Findoutmore: | extranet.who.int |
References: | Information provided with kind permission of WHO Global database on the Implementation of Nutrition Action (GINA): https://extranet.who.int/nutrition/gina/en |
Italian Society for Obesity (SIO) management algorithm for overweight or obese patients
A therapeutic algorithmic chart based on the well-established EOSS (Edmonton Obesity Staging System) composed of five stages. Uses colour coding to identify appropriate treatment options depending on stage of EOSS, BMI category and age group.
Categories: | Evidence of Management/treatment guidelines |
Year(s): | 2016 (ongoing) |
Target age group: | Adults and children |
Organisation: | Italian Society for Obesity (SIO) |
Findoutmore: | www.ncbi.nlm.nih.gov |
Linkeddocument: | Download linked document |
References: | Santini, F, Busetto, L, Cresci, B. and Sbraccia, P. (2016). SIO management algorithm for patients with overweight or obesity: consensus statement of the Italian Society for Obesity (SIO). Eating and Weight disorders. 2016(21). pp. 305307. |
European Union (EU) Action Plan on Childhood Obesity2014-2020
EU Action plan. To contribute to halting the rise in overweight and obesity in children and young people (0-18 years) by 2020
National Health Plan
Italian national health plan, including national obesity strategy
The Class Sports (Sport di Classe) programme
Class Sport is a project to promote physical education from primary school onwards.
Categories: | Evidence of Physical Activity Guidelines/Policy |
Year(s): | 2014 (ongoing) |
Target age group: | Children |
Organisation: | Ministry of Education, Universities and Research, the Italian Olympic Committee (CONI) and the Presidency of the Council of Ministers |
Findoutmore: | www.progettosportdiclasse.it |
Intensive Lifestyle Intervention to Treat Overweight/Obese Children and Adolescents
A multidisciplinary intervention including nutrition, exercise, and psychological aspects based on a family-based approach which was delivered for six months for children and three months for adolescents. The intervention aimed to assess the effects of participants' risk of cardiometabolic disease, changes in body composition, adherence to a Mediterranean diet and physical performance.
Categories: | Evidence of Multidisciplinary Intervention |
Year(s): | 2013-2014 |
Target age group: | Children |
Organisation: | Ranucci et al |
Findoutmore: | www.ncbi.nlm.nih.gov |
Linkeddocument: | Download linked document |
References: | Ranucci et al. 2017. Effects of an Intensive Lifestyle Intervention to Treat Overweight/Obese Children and Adolescents. Biomed Res Int. doi: 10.1155/2017/8573725. |
Evaluation of the EU school milk and fruit scheme
European Court of Auditors (2011) Are the school milk and school fruit schemes effective? Special Report No 10. Publications Office of the European Union, Luxembourg.
Categories: | Health Effectiveness Reviews (obesity related) |
Year(s): | 2011 (ongoing) |
Target age group: | Children |
Organisation: | EUROPEAN COURT OF AUDITORS |
Linkeddocument: | Download linked document |
References: | Information provided with kind permission of World Cancer Research Fund International from their NOURISHING framework www.wcrf.org/NOURISHING |
Healthy Stadia Network
"At Healthy Stadia, we encourage professional and amateur sports clubs, league operators, national and international governing bodies of sport to develop their stadia and sports facilities as health-promoting environments. We do this by supporting them to adopt a range of policies and practices in support of the health of their fans, their staff and the surrounding community. The definition we give to Healthy Stadia is: Healthy Stadia are those who promote the health of visitors, fans, players, employees and the surrounding community places where people can go to have a positive, healthy experience playing or watching sport."
Gaining Health - Guadagnare salute
This is a coordinated action plan for counteracting the 4 leading risk factors for noncommunicable diseases, including poor nutrition.
A multidisciplinary weight loss intervention in overweight and obese children and adolescents
A multi-disciplinary weight loss intervention aimed at reducing overweight and obesity in children and adolescents. This intervention involved medical, psychological and nutritional sessions, during which counselling on how to modify lifestyle choices was delivered.
Categories: | Evidence of Multidisciplinary Intervention |
Year(s): | 2006-2016 |
Target age group: | Children |
Organisation: | Mameli et al 2017. |
Findoutmore: | www.ncbi.nlm.nih.gov |
Linkeddocument: | Download linked document |
References: | Mameli et al 2017. Effects of a multidisciplinary weight loss intervention in overweight and obese children and adolescents: 11 years of experience. PLoS One. 2017; 12(7): e0181095. |
EU health and nutrient claims regulation
Regulation n 1924/2006 establishes EU-wide rules on the use of specified health and nutrient claims. The European Commission approves claims provided they are based on scientific evidence and accessible consumers. The European Food Safety Authority is responsible for evaluating the scientific evidence supporting health claims. Nutrition claims may only be used on food defined as "healthy" by a nutrient profile.
IDEFICS - Identification and prevention of Dietary- and lifestyle-induced health EFfects In Children and infantS
"A community-oriented intervention programme for primary prevention of obesity in a controlled study design. This intervention part of the IDEFICS study examined feasibility, effectiveness and sustainability of a coherent set of intervention modules addressing diet, physical activity and coping with stress."
Nutrition-Friendly Schools Initiative (NFSI)
"The NFSI is a school-based programme that addresses the double burden of malnutritionundernutrition, including micronutrient deficiencies, and overweight/obesity. It is a tool fordeveloping a school environment that promotes the nutritional well-being of school-agechildren and increases their physical activity levels."
Weight loss intervention
Counselling on lifestyle changes in small groups attending the clinic (advice on healthy eating, exercise, and reducing screen time).
Categories: | Evidence of Multidisciplinary Intervention |
Year(s): | 2006-2016 |
Target age group: | Children |
Organisation: | Endocrinology and Diabetes Clinic of V. Buzzi Children's Hospital |
Findoutmore: | journals.plos.org |
Linkeddocument: | Download linked document |
References: | Mameli, C, Krakauer, J.C, Krakauer, N.Y, Bosetti, A, Ferrari, C.M, Schneider, L, Borsani, B, Arrigoni, S, Pendezza, E, Zuccotti, G.V. (2017) 'Effects of a multidisciplinary weight loss intervention in overweight and obese children and adolescents: 11 years of experience' PLOSone, 12(7), https://doi.org/10.1371/journal.pone.0181095. |
Guidelines for healthy Italian food habits
These guidelines aim to establish and disseminate the basic information for a balanced diet.
Categories (partial): | Evidence of Nutritional or Health Strategy/ Guidelines/Policy/Action plan |
Year(s): | 2003 (ongoing) |
Target age group: | Adults and children |
Organisation: | Ministry of Health |
Findoutmore: | sito.entecra.it |
Linkeddocument: | Download linked document |
References: | National Research Institute on Food and Nutrition |
Code of Marketing Communication Self-Regulation
The self-regulatory organisation for the advertising industry, the Istituto dell Autodisciplina Pubblicitaria (IAP) has a Code of Marketing Communication Self-Regulation (1966, 47th edition effective January 16th, 2009) which includes a section on children and young people and mentions food. 59th edition - 2015.
Categories: | Industry/Government regulations - voluntary /pledges |
Year(s): | 1966 (ongoing) |
Target age group: | Adults and children |
Organisation: | The self-regulatory organisation for the advertising industry, the Istituto dell Autodisciplina Pubblicitaria (IAP) |
Findoutmore: | www.iap.it |
Linkeddocument: | Download linked document |
Giocampus
The Giocampus project is a multi-areas and multi-partner nutrition and sport educational program for children aged from 6 to 14 years held at the University of Parma in Italy. It joins physical activities, nutrition education, play and expressive and creative activities.
Categories: | Evidence of Community Interventions/Campaign |
Target age group: | Children |
Organisation: | The program is supported by the Town Council, School Inspectorate, Postgraduate School of Paediatrics, Graduate school of Sport and Exercise Sciences of University, Sport Clubs, the food industry and the media. |
Findoutmore: | www.giocampus.it |
GNPR 2016-17 (q7) Breastfeeeding promotion and/or counselling
WHO Global Nutrition Policy Review 2016-2017 reported the evidence of breastfeeding promotion and/or counselling (q7)
Categories: | Evidence of Breastfeeding promotion or related activity |
Target age group: | Adults |
Organisation: | Ministry of Health (information provided by the GINA progam) |
Findoutmore: | extranet.who.int |
References: | Information provided with kind permission of WHO Global database on the Implementation of Nutrition Action (GINA): https://extranet.who.int/nutrition/gina/en |
Grow Felix Project
"GROW FELIX" a project promoted by the Campania Regional Health Department, Campania Regional School Office...(which) addresses the issue of promoting proper nutrition and adequate physical activity with didactic itineraries specifically outlined for primary schools"
Standard Italiani per la Cura dell Obesita SIO-ADI 2016-17
Obesity Guidelines covering both adults and children in terms of Adults and Children_x000D__x000D__x000D__x000D_Prevention, Classification, Diagnosis, Treatment
Categories: | Evidence of Management/treatment guidelines |
Categories (partial): | Evidence of National Obesity Strategy/Policy or Action plan |
Target age group: | Adults and children |
Organisation: | (SIP) Societa Italiana di Pediatria (SINut) Societa Italiana di Nutraceutica(SISDCA) Societa Italiana per lo Studio dei Disturbi del Comportamento Alimentare |
Findoutmore: | www.sio-obesita.org |
Linkeddocument: | Download linked document |
References: | i Standard Italiani per la Cura della Obesita SIO-ADI 2016-17. (SIP) Societa Italiana di PediatriaSINut), Societa Italiana di Nutraceutica, (SISDCA) Societa Italiana per lo Studio dei Disturbi del Comportamento Alimentare |
The Walking bus - PIEDIBUS scheme
A scheme that promotes physical activity by walking to school.
Categories: | Evidence of Community Interventions/Campaign |
Target age group: | Children |
Organisation: | The scheme is run by the municipalities, local health authorities, schools and parents associations. |
Findoutmore: | www.piedibus.it |
No actions could be found for the above criteria.
The economic burden of obesity in Italy: a cost-of-illness study
Introduction
Obesity is a multifactorial health disorder characterised by an excessive accumulation of body fat, leading to a significantly increased risk for several chronic diseases, such as diabetes, cardiovascular diseases (CVDs), depression and cancer [15]. It has also been associated with a 520-year decrease in life expectancy [6, 7]. The prevalence of obesity has been rising worldwide in the past 50years, to the point of being widely recognised as an obesity pandemic [7, 8] with recent estimations of the Global Burden of Disease (GDB) reporting nearly a third of the world population currently classified as overweight or obese [6, 7]. The treatment of obesity and related comorbidities is projected to cost on average 8.4% of the total healthcare expenditure worldwide, with the United States (US) employing nearly 14% of their healthcare budget on obesity and overweight [9]. If the current trend continues unchanged, many European countries are also projected to have an obesity prevalence of 20% or more by 2025, with dramatic consequences on healthcare [10]. Detrimental effects have also been described in the work environment (i.e. sick leave, reduced productivity, and reduced employment), where obesity is projected to cause the loss of the equivalent of 6 million full-time workers by 2050 [9, 11].
In the context of this obesity pandemic, the situation in Italy is not very different. In the past decades, Italy was a symbol of a healthy lifestyle, with an obesity rate of only 8% of the population versus 30% in the US and 21% in the United Kingdom (UK). This rate was significantly lower than that in other Western countries as well, as reported in 2000 by the Organisation for Cooperation and Economic Development (OECD) [12]. However, between 1980 and 2013, the overweight and obesity rate in Italy increased by 27.5% and 47.1% in adults and children, respectively, likely due to lifestyle changes in favour of high-caloric foods and sedentary behaviours [13, 14]. The Italian Government attempted population- and individual-based interventions to contain and reverse this public health issue. For example, the Italian Health Ministry adhered to the Joint Action on Nutrition and Physical Activity (JANPA) initiative promoted by the European Union (EU) as part of a multi-project action aimed to halt the increase in overweight and obesity in children and adolescents by 2020 [15]. In addition, patients with a high body mass index (BMI), BMI40kg/m2 or BMI35kg/m2 in the presence of other comorbidities, became eligible for weight-loss surgeries fully reimbursed by the Italian National Health System (SSN) [16]. However, the interventions implemented by the Italian government did not seem to result in the desired effects, and latest national reports warn of a constantly increasing trend of obesity in both adults and children [13, 17, 18]. A major factor in this failure is represented by the massive investment directed by the food industry towards advertising food rich in sugar, fats and preservatives that promote obesogenic behaviours across adults and especially youngsters [19]. Moreover, a recent study reported both patients and physicians in Italy having a wrong perception of obesity. While obesity has been officially declared as a chronic progressive disease by the World Obesity Federation [20, 21], only 54% of obese people in Italy seek medical help, and only 36% receive a diagnosis [22].
Accurate measures on the economic burden of obesity are essential for raising awareness around this issue and eventually for developing effective public health interventions to address it. A valid tool to attempt the quantification of these costs is represented by cost-of-illness (COI) studies; economic studies aimed to identify, measure and report in monetary terms all costs that result from a specific disease [23]. COI studies quantify direct costsdirectly attributable to patient care such as costs for hospitalisations and drugs, as well as indirect costs, accounting for expenses that do not directly involve patients care, but that nevertheless impact society, such as costs for productivity losses or premature mortality [24, 25].
The utilisation of healthcare resources and the resulting attributable costs can be estimated in a COI study by adopting a topdown approach that uses aggregate data, or a bottomup approach that quantifies the health inputs used to produce specific healthcare services, and subsequently estimates the unit costs [24, 26]. Moreover, based on how epidemiological data are used in the study, COI studies can be divided into prevalence and incidence-based. The prevalence-based approach estimates the total costs of a disease incurred in a given year, and it is the most commonly used. The incidence-based approach implies the calculation of lifetime costs of patients diagnosed in a year of reference, thus providing a baseline against which new interventions can be evaluated [24].
While COI studies are effective tools in assessing the burden of disease, quantifying the accurate direct and indirect costs of obesity remains a complex task due to its multifactorial nature [27, 28]. An Italian COI study used estimates based on prescriptions from general practitioners (GPs) in relation to the BMI of their patients and portrayed a 30%-increase in the healthcare resource utilisation in the Italian obese population [29]. In 2012, a study estimated that only direct medical costs of obesity were responsible for 4% of the total healthcare expenditure in Italy, for a total of 4.5 billion projected to rise in the future [13, 17]. Similarly, a multi-country COI study evaluated the costs of the most common comorbidities of obesity in other European countries (Germany, the Netherlands and the Czech Republic) and estimated a 24% total healthcare expenditure attributable to overweight and obesity [30].
Even if the national and international literature already provides information on the magnitude of the obesity burden, the current knowledge does not yet offer an accurate description and projection of the phenomenon for Italian policy-makers [14, 28]. Specifically, most published COI studies on obesity present heterogeneous methodologies and different strategies when accounting for comorbidities or cost categories in their economic analysis, thus hindering the comparability of results across studies [28]. Another major challenge for the evaluation of the burden of obesity in Italy is represented by a marked discrepancy in data of prevalence of BMI classes, especially when comparing databases that collected self-reported data on obesity with databases that collected measured data [14]. For example, according to a report developed by the Italian Institute for Statistics (ISTAT) (multi-purpose analysis) in 2016 on self-reported data, the prevalence of obesity in Italy was 10% [31]. However, a study conducted using an Italian general practice registry, which collected measured data on the distribution of BMI classes, reported a prevalence of obesity of 22% [29].
At present, the national literature on the cost of obesity does not provide sufficiently homogeneous and comparable data to reach a global consensus. Therefore, the present study aims to estimate the economic burden of obesity in Italy, and thus support policy-makers in designing new strategies directed to tackle this constantly growing public health threat.
Methods
Study design
A quantitative COI study was conducted to assess the economic burden of obesity in Italy, adopting a societal perspective which considers direct healthcare costs and productivity losses [32]. This study adopted a topdown (population-based) approach, using aggregate data on mortality, morbidity and other disease-related costs and indicators [33]. Costs were calculated using a prevalence-based approach, in which the costs associated with a disease are calculated on an annual basis [34]. After identifying data on the prevalence of obesity and prevalence of obesity-associated comorbidities, costs were estimated using the Population Attributable Fraction (PAF), which represents an estimate of the percentage of the patient population (for each pathology) is attributable to obesity (exposure) and highlights the causal link between the exposure and the attributable pathology [35]. At present, Italy lacks a country-specific check-list for the development and quality assessment of COI studies. Thus, a check-list developed by the Health Economics group of the German Network for Healthcare Research has been used as a methodological guideline [36].
Search of data on obesity prevalence and validation of input data sources
In this study, obesity is defined as having a BMI30kg/m2, as indicated by the Centre for Disease Control and Prevention [37]. Data on the prevalence of obesity in Italy was obtained by searching the international and national literature with a snow-ball method starting from two national reports on obesity [17, 38]. The included sources fulfilled the following inclusion criteria: (1) investigation of prevalence of overweight (BMI25 to<30kg/m2) and obesity (BMI30kg/m2), (2) inclusion of data on the Italian population, (3) reporting of BMI measurements for male and female adults (18years old). Databases that focussed on the prevalence of obesity in children and adolescents were excluded. The extraction of database characteristics was carried out before data quality assessment and included: name of the database, year of publication, sample size, study population age range, adopted definition of adult age, data collection method and BMI classification and data on prevalence (mean value, 95% Confidence Interval). Each measure was double-checked, consulting the original source and extracting values from disaggregate data when possible. If data were provided as stratified by gender, the value was manually derived by calculating the weighted average. Nine criteria based on the methodology developed by the Essnet Validat Foundation [39] were used for the quality assessment of the sources. After extracting the database characteristics, a point was assigned for each of these items: source reliability, data completeness (time frame, geographical area, included variables), inclusion of all BMI classes, reporting the sample size for Italy, clear methodology, consistency of results with other sources, use of the data in previous research, endorsement of a field expert. Two points were assigned if measured data were collected, as previous research highlighted the underestimation of obesity prevalence when collecting self-reported data [4042]. Supplementary Table 1 reports the original definition of validation and quality criteria for external data sources as described by the Essnet Validat Foundation [39]. The source with the highest score according to the validation tool was chosen for the analysis.
Bariatric surgery
The weight-loss or bariatric surgery is a primary component in determining costs associated with obesity. According to the guidelines of the Italian Society for Bariatric Surgery (SICOB), patients with a BMI40kg/m2 (class III obesity) or a BMI35kg/m2 (class II obesity) in the presence of other comorbidities are eligible for a bariatric surgery that is fully paid by the Italian National Health System (SSN) [16]. For this study, the prevalence of class II and class III obesity was retrieved from the literature. However, only 1.4% of eligible patients received on average bariatric surgery in Italy, as reported by the Italian report Osservatorio PariSanit [43], which was the value used for our cost calculation.
Obesity-associated pathologies, relative risks and population attributable fractions
Obesity is a major risk factor for chronic diseases such as metabolic disorders, CVDs, and different types of cancer, which contribute to the overall economic burden of obesity [14, 4446]. In this study, only the most common obesity-associated pathologies were included, namely diabetes and CVDs (angina, atrial fibrillation, cerebrovascular diseases, congestive heart failure, hypertension, ischaemia, myocardial infarction, pulmonary embolism, and stroke). In addition, 11 types of cancer (breast, colon-rectum, kidney, leukaemia, liver, oesophagus, ovaries, pancreas, prostate, thyroid, and uterus) were included, as increasing evidence highlighted the role of obesity in cancer development [6, 47]. They, therefore, represent an interesting case, to date scarcely explored in Italy. Only costs associated with CVDs, diabetes, and cancer were included in the study as they offer an exhaustive measure of the burden of obesity-associated pathologies, with other comorbidities causing negligible costs in comparison [29, 38].
Data on the prevalence of these pathologies were retrieved by conducting a snow-ball literature review in MEDLINE (PubMed) and Embase. Next, the relative risk (RR) attributable to obesity for each pathology was identified. The RR indicates the ratio between the probability of an outcome in the exposed group (with obesity) and the probability of the same outcome in an unexposed group (without obesity) [48]. When data specific for Italy were not available, the RRs were retrieved from studies conducted in countries comparable to Italy in terms of environmental, social, and economic dimensions. The RRs were used to calculate the PAF for each pathology to identify which percentage of the patient population is attributable to obesity (exposure) [35]. The PAF indicates a causal relationship between the exposure and pathology, indicating in this study, not only the population affected by both obesity and another pathology simultaneously but also the one that developed the pathology exclusively because of obesity. The PAF for an obesity-associated pathology i was calculated as follows:
where Po denotes obesity prevalence and RRi denotes relative risk of the obesity-associated pathology i.
The fraction of the population PCO with a pathology i due to obesity was calculated multiplying the total population affected by that pathology PCi by the PAF of that pathology PAFi as follows:
Cost evaluation
Direct costs
The cost evaluation considered both direct costs solely attributable to obesity (i.e. bariatric surgery) and direct costs attributable to obesity-associated pathologies. Cost categories were searched considering all possible direct costs for obesity and obesity-attributable pathologies (i.e. drugs, nursing services, and physician visits). Based on the results of the literature search, only the items relevant to obesity were included in the cost calculation, namely costs for drugs, hospitalisations, monitoring and adverse events. More specifically, hospitalisation costs included costs for primary care, inpatient care, and outpatient care.
The total costs of bariatric surgery were estimated using the most recent Italian estimates from the Italian report Osservatorio PariSanit, that reported pro-capita average costs of bariatric surgery in Italy, taking in account acute care, long-term care and rehabilitation [43]. Costs pro-capita were multiplied by the number of class II and class III obese patients, that are eligible for the surgery according to the SICOB guidelines [16]. The Italian rate of patients receiving the surgery on the total eligible population, namely 1.4% [43], was considered for the cost calculation.
The costs attributable to each obesity-associated pathology were estimated by identifying the pro-capita costs in the literature. The PCO was multiplied by the costs pro-capita DCi to obtain total healthcare costs TCi per year for each pathology as follows:
In addition, the MEDLINE (PubMed) and Embase databases were searched using a snow-ball method to obtain data on costs of weight-loss programmes, psychological counselling, and special transportation (i.e. bariatric ambulance).
When needed, costs have been adjusted to 2020, using the CCEMG-EEPI-Centre cost converter, a web-based tool recommended by the World Health Organisation (WHO) to adjust an estimate of costs to a target currency and/or price year [49]. To minimise the risk of double counting when extracting cost items from the literature, the lowest cost per person in the absence of other comorbidities was included. Alternatively, cost categories were filtered out to select the items relevant to each obesity-associated pathology.
Indirect costs
The MEDLINE (PubMed) and Embase databases were searched using a snow-ball method to obtain information on productivity loss due to obesity. The cost items specific for Italy available in the literature, namely costs for presenteeism and absenteeism, were included in the calculation of obesity-attributable costs. The average costs pro-capita were extracted from the study by Gupta et al. [50] that adopted the human capital approach to estimate the productivity losses. After identifying the average cost per person, the costs reported for normal-weight individuals were subtracted from those reported for the obese population to obtain obesity-attributable costs. The cost difference was multiplied by the number of obese people to obtain costs at a population level. Costs were adjusted to 2020 using the CCEMG-EEPI-Centre cost converter.
Sensitivity analyses
A deterministic (one-way) sensitivity analysis was conducted to address the uncertainty of the data included in the model and to validate the study results. The minimum and maximum values of all the variables included in the model were defined by increasing or decreasing each item value by 10%. Seven variables were tested in the deterministic sensitivity analysis, namely obesity prevalence, rate of eligible patients receiving a bariatric surgery, total costs of bariatric surgery, total costs of obesity-associated CVDs, total costs of obesity-associated diabetes, total costs of obesity-associated cancer, total costs of obesity-associated productivity losses. In particular, the minimum and maximum rates of patients that received the bariatric surgery were set at 1% and 3%, respectively, with the purpose of simulating a similar trend to the ones previously reported for other countries [51, 52].
In addition, a probabilistic sensitivity analysis (PSA) for the base case was performed, adopting the Monte Carlo method (second order) and replicating the calculation of the total obesity costs with 1,000 simulations. The estimated values for the PSA were based on the assumed value distribution, point estimates and standard error (SE).
Results
Data on prevalence of obesity and validation of input sources
As mentioned above, a purposive literature review was conducted to identify data on prevalence of obesity in Italy. Data on prevalence were extracted from the most recent population-based databases that investigated obesity in the Italian adult population and summarised in Table . In particular, the search identified seven national and international databases, whose main characteristics, namely source and year of data publication, data collection (measured or self-reported), sample size and BMI classification definition are reported in Supplementary Table 2. While the age range of the study populations differed markedly across databases (Supplementary Table 2, Age Range), the definition of BMI classes was overall consistent and included four main categories: underweight, normal-weight, overweight and obese. However, the Health Search database further stratified obese patients in class I (BMI30 to<35kg/m2), class II (BMI35 to<40kg/m2) and class III (BMI40kg/m2) [53]. If prevalence measures were only provided as stratified by gender, the data were manually derived, calculating the weighted average (Table , data marked with an asterisk (a)). The complete prevalence data, including details on less recent reports, confidence intervals (CI 95%), gender of the participants and public accessibility, were reported in Supplementary Table 3.
Table 1
Prevalence data for body mass index (BMI) classes (obesity, overweight, normal, and underweight) for adults in Italy from seven different databases
Database source and year | Data collection | UnderweightBMI<18.50 (%) | Normal18.50BMI>25 (%) | Overweight25BMI>30 (%) | ObeseBMI30 (%) |
---|---|---|---|---|---|
Health Search, 2010 [29] | Measured | 2.66 | 40.8 | 36.88 | 19.66a |
Health Search, 2012 [53] | Measured | 2.3 | 38.1 | 37.4 | 22.2b |
Progetto CUORE, 2012 [18, 44] | Measured | 40.5a | 25.8a | ||
Global Burden of Disease, 2013 [6] | Mixed | 49.9a | 18.2a | ||
Global Burden of Disease, 2015 [64] | Mixed | 34a | 11a | ||
EUROSTAT (EHIS), 2015 [54] | Self-reported | 3.3 | 51.9 | 34.1 | 10.8 |
Global Health Observatory, 2016 [92] | Measured | 0.8 | 35.1a | 41.2a | 22.9 |
ISTAT multipurpose, 2018 [93] | Self-reported | 3 | 50.26 | 35.76 | 10.98 |
Indagine Passi, 2018 [94] | Self-reported | 3.1a | 54.4a | 31.60 | 10.9 |
The seven databases presented similar values for the prevalence of underweight individuals in the Italian population (~2.6%). On the contrary, prevalence of overweight and obesity differed markedly, with obesity ranging from 10.8% as reported by EUROSTAT [54] to 25.8% as reported by Progetto CUORE (OEC/HES) [18]. Similar values were displayed by databases that collected self-reported (~10.5%) or measured data (~20%). The GBD study performed in 2015 reported similar values to the ones by ISTAT, EUROSTAT, and PASSI, albeit using both self-reported and measured data.
Due to the heterogeneity of these sources, an input data validation was performed to choose the source to be included in the analysis. Nine validation criteria were adapted from the methodology proposed by the Essnet Validat Foundation [39] (see Methods section). The validation matrix and the original criteria formulation are reported, respectively, in Supplementary Tables 1 and 4. The Health Search database was the only source that obtained a full score and its most recent measures (2012) were therefore selected for the analysis (see Supplementary Table 5). The Health Search measures on prevalence of obesity, stratified according to age group and BMI index, are reported in Table .
Table 2
Prevalence of obesity in Italy stratified by age and BMI classification,
adapted from Colao et al. [53]
Prevalence | Normal weight18.50BMI>25, (%) | Overweight25BMI>30, (%) | ObeseBMI30, (%) | Total (%) |
---|---|---|---|---|
1829years | 62.0* | 22.3* | 15.8* | 100 |
3064years | 40.8** | 36.4** | 22.8** | 100 |
65+years | 32.1 | 44.2 | 23.7 | 100 |
Bariatric surgery
The number of patients receiving a bariatric surgery was calculated using the prevalence of BMI classes from Colao et al. [53]. As data on prevalence referred to 2012, the Italian population in 2012 was taken from the ISTAT database (ISTAT 2012). The SICOB guidelines consider eligible to bariatric surgery only patients with a BMI40kg/m2 or BMI35kg/m2 in the presence of other comorbidities [16]. However, only 1.4% of eligible patients on average receive bariatric surgery, as reported by a recent national report [43]. Therefore, this share of the population was used for the cost analysis, resulting in the estimation of 41,880 bariatric surgery in 2020 in Italy, considering a prevalence of obesity of 22.2%.
Obesity-associated pathologies, relative risks and population attributable fractions
Key obesity-associated pathologies were included in the analysis, namely diabetes, CVDs and cancer (see Methods). Diabetes and CVDs were included as their impact on the overall burden of obesity has been widely described in the literature [17, 29, 55]. Moreover, 11 types of cancer were selected and included in the analysis, as increasing evidence described their connection with obesity [5659] and thus represented a case of specific interest. Only the cancer types whose risk has been associated with obesity were included.
To estimate the cases of diabetes and CVDs attributable to obesity (Table ), it was necessary to first calculate the number of total cases in Italy. Figures on the prevalence of diabetes and CVDs were obtained from Atella et al. [60], Giampaoli et al. [44] and Moretti et al. [61] (Supplementary Table 6) and multiplied by the Italian adult population of the corresponding year. All data on prevalence referred to 2014, except for cerebrovascular diseases (prevalence in 2012) and pulmonary embolism (prevalence in 2007). Therefore, the Italian population in 2014, 2012 and 2007 was taken from the ISTAT database (ISTAT 2007, 2012, 2014).
Table 3
Obesity-associated cases of cardiovascular diseases (CVDs) and diabetes in Italy estimated using the Population Attributable Fraction (PAF)
Disease | Populationa | Prevalence (%) | Cases in Italy | RRb | PAF (%) | Cases due to obesity |
---|---|---|---|---|---|---|
Cardiovascular diseases | ||||||
Angina | 43,054,180 | 0.8 | 344,433 | 1.96W | 18 | 60,510 |
Atrial fibrillation | 43,054,180 | 2.9 | 1,248,571 | 1.49Wa | 10 | 122,495 |
Cerebrovascular disease | 38,056,749 | 0.7 | 266,397 | 1.54W | 11 | 28,517 |
Congestive heart failure | 43,054,180 | 1.3 | 559,704 | 1.79G | 15 | 83,514 |
Hypertension | 43,054,180 | 30.0 | 12,916,254 | 2.41GBD | 24 | 3,079,196 |
Ischaemic heart disease | 43,054,180 | 3.5 | 1,506,896 | 2D | 18 | 273,757 |
Myocardial infarction | 43,054,180 | 1.0 | 430,542 | 1.44W | 9 | 38,313 |
Pulmonary embolism | 36,548,850 | 0.02 | 6908 | 3.51G | 36 | 2472 |
Stroke | 43,054,180 | 4.9 | 2,109,655 | 1.56D | 11 | 233,272 |
Endocrinological diseases | ||||||
Diabetes | 43,054,180 | 8 | 3,444,334 | 6.25D | 54 | 1,853,785 |
Next, it was necessary to identify the RRs that in the case of obesity indicate the risk for an obese person to develop a certain pathology compared to a normal-weight person. The RRs were extracted from five studies, selecting when possible measured data from European study populations: the DYNAMO-HIA Project, a European report, which includes a systematic review and meta-analysis of RR linked to obesity [62]; the systematic review and meta-analysis of Guh et al. [47] of US (55%) and European studies (40%); the cohort study by Wilson et al., [46] conducted in the US with about 5200 participants; the meta-analysis of Wanahita et al. [63]; and the Global Burden of Disease, which investigated the burden of obesity worldwide [64].
After identifying the RRs for each considered pathology, the PAF was calculated as described in the methods, to estimate which fraction of the population affected by a pathology is attributable to obesity. The calculations performed to obtain the PAF for each obesity-associated pathology are reported in detail in Supplementary Table 7. A similar methodology was employed for estimating the obesity-associated cases of cancer reported in Table . However, data on prevalence of all the cancer types included in the study were not available in the literature. Therefore, the proportion of each cancer type from the total of cancer cases diagnosed in Italy in 2014 was obtained from the Associazione Italiana di Oncologia Medica (AIOM) report I numeri del cancro in Italia, 2014 [65] and multiplied by the total of cancer cases in 2014 to obtain the number of cases specific for each cancer type. Obesity-attributable cancer cases were then estimated using the PAF, as described for the other pathologies.
Table 4
Obesity-associated cases of cancer in Italy estimated using the Population Attributable Fraction (PAF)
Cancer type | Proportion (%)a | Cases in Italy | RRb | PAF (%) | Cases due to obesity |
---|---|---|---|---|---|
All | 100 | 2,243,953 | |||
Breast | 23.3 | 522,235 | 1.25D | 5 | 27,460 |
Colon-rectum | 13.2 | 296,687 | 1.25D | 5 | 15,600 |
Kidney | 3.8 | 84,413 | 1.68D | 13 | 11,072 |
Leukaemia | 2.3 | 51,378 | 1.11GBD | 2 | 1225 |
Liver | 1.0 | 21,416 | 1.24GBD | 5 | 1083 |
Oesophagus | 0.2 | 3700 | 2.3D | 22 | 829 |
Ovaries | 1.7 | 37,829 | 1.04GBD | 1 | 333 |
Pancreas | 0.4 | 9636 | 1.08GBD | 2 | 168 |
Prostate | 9.7 | 216,716 | 1.05G | 1 | 2379 |
Thyroid | 3.6 | 81,129 | 1.18GBD | 4 | 3117 |
Uterus | 4.1 | 91,689 | 1.61GBD | 12 | 10,936 |
Cost evaluation
Direct costs
This analysis included obesity-attributable direct costs for drugs, hospitalisations, monitoring and adverse events. All costs referred to the year 2020; where needed costs have been adjusted using the CCEMG-EEPI-Centre cost converter (see Methods section) [49].
The costs of bariatric surgery were calculated using the costs pro-capita from the Italian report Osservatorio PariSanit, that reported an expense of 5779 per patient (Table ) [43]. To obtain the total costs of bariatric surgery, costs pro-capita were multiplied by the number of surgeries estimated in the year 2020, based on the requirements of SICOB guidelines [16] and the rate of patients receiving bariatric surgery in Italy [43] (Table ).
Table 5
Yearly average direct costs pro-capita for patients receiving a bariatric surgery or for patients affected by diabetes, cancer or cardiovascular diseases (CVDs), adjusted to 2020
Disease | Direct costs pro-capita | Reference |
---|---|---|
Bariatric surgery | 5779 | DAngela et al. [43] |
Diabetes | 353 | Marcellusi et al. [66] |
Cancer | 4489 | Jnsson et al. [73] |
Cardiovascular diseases | ||
Anginaa | 6066 | |
Atrial fibrillation | 3597 | Ringborg et al. [67] |
Cerebrovascular diseasea | 6066 | |
Congestive heart failure | 12,028 | Corrao et al. [68] |
Hypertension | 272 | Scholze et al. [69] |
Ischaemiaa | 6066 | |
Myocardial infarction | 11,584 | Mantovani et al. [70] |
Pulmonary embolism | 1496 | Gussoni et al. [71] |
Stroke | 7419 | Gerzeli et al. [72] |
Table 6
Obesity-attributable direct costs in millions of euros for bariatric surgery, cancer, cardiovascular diseases (CVDs) and diabetes, adjusted to 2020
Disease | Direct health costs due to obesity (mln) |
---|---|
Surgical procedures | |
Bariatric surgery | 242.0 |
Neoplasms of | |
Breast | 123.3 |
Colon-rectum | 70.0 |
Kidney | 49.7 |
Leukaemia | 5.5 |
Liver | 4.9 |
Oesophagus | 3.7 |
Ovaries | 1.5 |
Pancreas | 0.8 |
Prostate | 10.7 |
Thyroid | 14.0 |
Uterus | 49.1 |
Cardiovascular diseases | |
Angina | 367.1 |
Atrial fibrillation | 440.6 |
Cerebrovascular disease | 173.0 |
(Congestive) heart failure | 1004.5 |
Hypertension | 837.5 |
Ischaemia | 1660.6 |
Myocardial infarction | 443.8 |
Pulmonary embolism | 3.7 |
Stroke | 1730.6 |
Endocrinological diseases | |
Diabetes | 654.4 |
Total direct health costs | 7891.0 |
Patient costs pro-capita in Italy for the included obesity-associated pathologies were taken from published studies and summarised in Table . When possible, the lowest cost pro-capita estimated in the absence of other comorbidities was taken to minimise the double count of pathology-attributable costs. However, the PAF used to estimate the obesity-attributable cases for each pathology implies a causal relationship exposure-outcome, thus already indicating the presence of more pathologies (obesity plus another one). Taking costs in the absence of comorbidities, and thus of non-comorbid patients, may result in an underestimation of costs.
Considering costs adjusted to 2020, Marcellusi et al. [66] reported a cost pro-capita of 353 for diabetes without comorbidities. Yearly direct costs were 3597 for atrial fibrillation [67], 12,028 for (congestive) heart failure [68], 272 for hypertension [69], 11,584 for myocardial infarction [70], 1496 for pulmonary embolism [71], and 7419 for stroke [72]. Costs pro-capita for angina, cerebrovascular diseases and ischaemia were not available in the literature. Therefore, the average cost of the six CVDs was calculated and used for further analysis. Similarly, costs specific for the included cancer types could not be found in the literature and were estimated using data from Jnsson et al. [73], which reported total direct costs in Italy for the year 2014. The total costs were divided by the number of cancer patients in Italy in 2014, assuming an equal average pro-capita cost for all cancer patients to obtain costs pro-capita. The number of obesity-attributable cases for diabetes, CVDs and cancer (Tables , ) was multiplied by the costs pro-capita reported in Table , to obtain the total obesity-attributable direct costs for each included pathology (Table ).
Healthcare costs for GP contacts attributable to obesity were excluded, as the studies that reported the volume utilisation for GP visits did not differentiate between visits attributable to obesity alone and to obesity-associated pathologies [74, 75]. Likewise, costs for weight-loss programmes, psychological support and special transportation (i.e. bariatric ambulance) due to obesity were excluded from this study, due to limited data availability in the literature.
Indirect costs
Obesity-attributable indirect costs include productivity lossesi.e. costs for presenteeism and absenteeismand have been adjusted to 2020 using the CCEMG-EEPI-Centre cost converter [49]. Costs pro-capita were taken from Gupta et al. [50], the only available study that provided costs for Italy. Their study included the EU5 (France, Germany, Italy, Spain and the UK) and estimated annual indirect costs using the human capital method, multiplying wages by the percentage of work productivity impairment [50]. Gupta et al. provided estimates as averages of the values obtained for the five included countries (3409 for normal-weight people; 3633 for class I obese, 4077 for class II obese, 5307 for class III obese) and address the heterogeneity across different population groups by exclusively considering costs for employable people. For the calculations here reported, obesity-attributable costs were obtained by subtracting the costs reported for normal-weight individuals from those reported for the obese.
Absenteeism and presenteeism costs were calculated separately and stratified for the three obesity classes (129, 415, 857 due to absenteeism in class I, II, III, respectively; 124, 372, 1,299 for presenteeism in class I, II, III, respectively). The number of obese employees was calculated using data on obesity prevalence in 2012 [53] on a total of 10,957,666 individuals (Italian adults in 2012, ISTAT). Costs pro-capita were multiplied by the number of obese employees, resulting in total indirect costs of 2.62 billion for absenteeism and 2.83 billion for presenteeism (Table ).
Table 7
Indirect costs (IC) due to obesity-attributable absenteeism (A) and presenteeism (P)
BMI Class | Prevalence (%) | Obese by BMI class | IC A | IC P | IC A due to obesity | IC P due to obesity | IC A/year (mln) due to obesity | IC P/year (mln) due to obesity |
---|---|---|---|---|---|---|---|---|
Normal weight (BMI<25) | 955 | 2709 | ||||||
Obese I (BMI 3035) | 72.8 | 7,977,181 | 1084 | 2833 | 129 | 124 | 1029 | 986 |
Obese II (BMI 3540) | 20.1 | 2,202,491 | 1369 | 3081 | 415 | 372 | 913 | 819 |
Obese III (40BMI>70) | 7.2 | 788,952 | 1812 | 4008 | 857 | 1,299 | 676 | 1025 |
All classes | 100 | 10,957,666 | 2618 | 2830 |
Overall costs
The overall costs attributable to obesity in 2020 for Italy are reported in Table and amounted to 13.34 billion, with 0.24 billion for bariatric surgery, 0.65 billion for diabetes, 6.66 billion for CVDs, 0.33 billion for cancer and 5.45 billion losses of productivity. Direct healthcare costs represented 59.2% of the overall costs, while indirect costs accounted for the 40.8%.
Table 8
Costs attributable to obesity in billions of EUR in Italy in 2020
Direct healthcare costs | ||
Bariatric surgery | 0.24 | 1.8% |
Diabetes | 0.65 | 4.9% |
Cardiovascular diseases | 6.66 | 49.9% |
Cancer | 0.33 | 2.5% |
Total direct costs | 7.89 | 59.2% |
Indirect costs | ||
Absenteeism | 2.62 | 19.6% |
Presenteeism | 2.83 | 21.2% |
Total indirect costs | 5.45 | 40.8% |
Total | 13.34 | 100.0% |
Sensitivity analysis
The model results were validated by performing a deterministic (one-way) sensitivity analysis that tested seven variables: (1) obesity prevalence, (2) total costs of bariatric surgery, (3) total costs of obesity-attributable CVDs, (4) total costs of obesity-attributable diabetes, (5) total costs of obesity-attributable cancer, (6) total costs of obesity-associated productivity losses, and (7) rate of eligible patients receiving bariatric surgery. Results indicated the minimum and maximum total obesity costs when one of the above variables (parameters) changed through a predefined minimummaximum bond. The results are reported in a tornado diagram that displays the variation in the overall costs of obesity when varying each of the above variables (parameters) chosen for the deterministic sensitivity analysis (Fig.).
Deterministic (one-way) sensitivity analysis and tornado diagram. Seven variables were tested to address uncertainty of the following parameter values when estimating the economic burden of obesity: (1) obesity prevalence, (2) total costs of bariatric surgery, (3) total costs of obesity-attributable CVDs, (4) total costs of obesity-attributable diabetes, (5) total costs of obesity-attributable cancer, (6) total costs of obesity-associated productivity losses, and (7) rate of eligible patients receiving bariatric surgery. Parameter values are changed through upper and lower bounds to estimate minimum and maximum total obesity costs
The prevalence of obesity was the variable that showed the relatively highest variation in total obesity cost, resulting in a minimum of 12.13 billion when reducing the obesity prevalence by 10% and a maximum of 14.52 billion when increasing it by 10%. Total indirect costs and bariatric surgery rate had a relatively minor impact on total obesity cost, determining a variation of plus/minus 33.3 and 24.20 million, respectively, when taking their minimum or the maximum value in this study.
In addition, the results were validated by performing a PSA (Fig.). After running 1,000 simulations of the model, the total costs of obesity displayed a 95% credible interval of 8.99 billion<<17.80 billion.
Probabilistic sensitivity analysis (PSA) performed to address uncertainty of parameter values when estimating the total burden of obesity. The PSA was performed adopting the Monte Carlo method (second order) and calculation of the total obesity costs was replicated with 1,000 simulations
Discussion
This study aimed to estimate the direct and indirect costs attributable to obesity in Italy in 2020. The first challenge of this research was to choose reliable estimates of the obesity prevalence in the country. In Italy, there is currently no real consensus on the prevalence of obesity (BMI30kg/m2) which, according to the different sources, ranged between 10.8 [54] and 25.8% [18]. The most evident reason for this variation appeared to be the adopted data collection method. Studies that collected self-reported data reported on average~12% less obese than the studies using measured data, thus underestimating the magnitude of the problem. This fact is supported by previous research confirming the underestimation of obesity prevalence when using self-reported data [4042]. The present study used data from the Health Search database, which estimated an obesity prevalence of 22.2% (obesity class I: 72.8%; class II: 20.1%; class III: 7.2%) using measured data (Table ) [53].
As the findings of the literature search did not show any Italian databases that provide data on obesity and contemporarily on all the obesity-associated pathologies included in the study, aggregate measures were retrieved from the literature (topdown approach). In addition, previous literature highlighted the advantage of adopting this approach when including multiple diseases in the economic evaluation. In fact, allocating the total country expenditures among the most important cost items minimises the risk that the sum of individual costs related to each included diseaseestimated by adopting a bottomup approachis greater than the total national health care expenditure and thus minimises the risk of duplicating costs [76].
Direct costs were determined considering the costs of bariatric surgery and the costs of obesity-associated pathologies as main components of the total obesity burden. Previous research widely described the association of obesity with diabetes and CVDs, which were thus included in the analysis (Table ). In addition, 11 types of cancer were considered due to the increasing evidence of their association with obesity [6, 47]. The contribution of cancer to the economic burden of obesity is currently scarcely explored in Italy and therefore represented a particularly interesting aspect for the present study. Direct costs amounted to 7.89 billion, the main drivers being CVDs (6.66 billion) that accounted for~49.9% of the overall costs, while the costs of bariatric surgery had the smallest impact on costs among the included cost items (0.24 billion).
Costs of hypertension and diabetes were 0.84 and 0.65 billion, respectively (Table ). These findings are in line with other studies that reported a similar impact of these two pathologies on the burden of obesity [29, 77]. Peculiar were the cases of ischaemia (1.65 billion) and stroke (1.72 billion), which doubled the costs of diabetes and hypertension (Table ), likely due to differences in the cost selection performed prior to the cost evaluation. On one side, costs pro-capita of ischaemia were calculated as an average of the other CVDs and costs of stroke were taken from a study that did not account for the presence of comorbidities, thus probably resulting in an overestimation of costs [72]. On the contrary, diabetes and hypertension costs were obtained from studies that differentiated costs in the presence/absence of comorbidities [66, 69]. In this case, the lowest value was selected in a prudential mode to minimise duplication of costs with other pathologies, which likely lead to an underestimation of costs. In fact, the PAF implies a causal relationship with obesity and another pathology, thus already defining the patients as comorbid. In addition, obese and severely obese patients are more likely to present multiple comorbidities interacting together. A severely obese patient affected by diabetes and hypertension will then result in a greater need for healthcare resources compared to two patients independently affected either by diabetes or hypertension.
Costs attributable to bariatric surgery amounted to 0.24 billion, representing the expenses that result from the treatment of 1.4% of the eligible patient population [43]. This rate indicates a severe under-treatment of obese patients needing weight-loss surgeries in Italy. However, the 1.4% rate is in line with the ones reported for other countries in Europe, such as Germany (less than 1%) [51, 78], and UK (0.002%) [52]. Italy displayed a significantly lower rate of bariatric surgeries compared to France and Belgium, probably due to different accessibility criteria and financial rules [51]. In France in 2018, for example, more than 30% of surgeries were performed on patients with a BMI<40kg/m2 and mostly in the absence of comorbidities [79].
Costs associated with GP visits, counselling programmes and special transportation (i.e. bariatric ambulance, which requires a supplemental price compared to a regular ambulance) were excluded due to the lack of data in the literature. For example, previous studies did not differentiate between GP visits attributable to obesity alone and obesity-associated pathologies [74, 75]. The exclusion of these categories resulted in underestimating direct costs. However, these costs are negligible when compared with the economic impact of pathologies such as diabetes or CVDs.
Indirect costs amounted to 5.45 billion (2.62 for absenteeism; 2.83 for presenteeism) (Table ). Costs pro-capita were taken from Gupta et al., which estimated the indirect costs of obesity in five countries, including Italy, using the human capital approach [50]. However, Gupta et al. did not report costs at a population level and thus did not allow a comparison with the results presented here. A recent review reported obesity-attributable productivity losses ranging from $89 to $1586 for absenteeism and $11 to $4175 for presenteeism, in line with the costs used in this analysis (average of 467 for absenteeism and 598 for presenteeism) [80]. Three German COI studies reported similar findings with the ones presented here: Effertz et al. estimated $4.97 billion of obesity-attributable indirect costs in 2016 [81], Konnopka et al. estimated 5 billion attributable to both overweight and obesity in 2002 [77], and Knoll et al. projected 3.6 billion of obesity-attributable indirect costs in 2020 [82]. The costs reported by Effertz et al. [81] and Konnopka et al. [77] represent, respectively,~1.4% and~2.2% of the German health expenditure for the years of reference [83, 84], compared to the 5.5 billion reported in this study, which account for~4.8% of the Italian health expenditure (considering the health expenditure of 2019) [85]. A COI study performed in Canada in 2006 by Klarenbach et al. estimated lower costs for absenteeism compared to this study ($187 million vs. 676 million for class III obese) [86]. However, Klarenbach et al. used self-reported data on BMI, which, as previously discussed, may have resulted in underestimating costs. Higher indirect costs were reported by studies performed in the USA, likely due to the differences in obesity prevalence compared to Italy. For example, Ricci and Chee estimated $11.7 billion, considering a 42% prevalence [87].
According to the findings reported here, the total burden of obesity in Italy for 2020 is 13.34 billion. These costs are higher than those proposed by Atella et al. in 2012, which estimated a burden of 4.5 billion adopting a population-based approach (bottomup) and using prevalence data from Health Search [17, 29]. A reason for this discrepancy could be the topdown approach of this study and the use of the PAF, which may have resulted in a duplication of costs. The COI study by Konnopka et al. estimated total obesity- and overweight-attributable costs of ~10 billion in 2002 in Germany [77]. If adjusted to 2020 the overall costs amount to~12 billion in line with the findings presented in this study.
The uncertainty of the data included in the model was addressed by performing a deterministic sensitivity analysis and a PSA (Figs., ). Varying the prevalence of obesity had the highest impact on the total obesity costs, determining a 2.4-billion reduction on overall obesity costs when the obesity prevalence was decreased by 10%. This result further underlined the key importance of accurate measures of prevalence in economic evaluations of obesity. Similarly, the costs of CVDs and diabetes had a major impact on overall obesity costs compared to the rate of bariatric surgery, the latter increasing the total costs of obesity by 350 million when doubled. The PSA returned a credible interval of 8.99 billion<<17.80 billion.
A major challenge that occurred when comparing results from similar previously published COI studies was the heterogeneity of contextual factors across studies, such as drug pricing, demographic characteristics of the population, and healthcare system organisation [88, 89]. Accounting for different contextual factors is particularly important when adopting a healthcare system perspective, for example in the context of a public or private healthcare system. It is therefore important to have studies that specifically address the burden of obesity in Italy, to compare results from studies that have similar contextual factors and tackle the challenge of data transferability.
The COI model developed in this study presents valuable strengths. A validation tool was developed to select the most reliable and accurate source for data on prevalence (Supplementary Table 5), as Italy currently lacks a univocal agreement on prevalence of obesity in the country. Adopting a validation tool ensured the selection of a source that satisfied a number of selection criteria describing completeness, accuracy, comparability and clarity of data. Another strength of the study is represented by the fact that the contribution of each obesity-associated pathology was evaluated separately (Table ), aiming to accurately portray the weight of each pathology. For example, Konnopka et al. grouped pathologies in categories (neoplasms, CVDs etc.) and assumed equal costs for diseases in the same category [77]. However, pathologies within a category may differ in costs, as for hypertension and stroke, which in the present study were associated with direct costs of 272 and 7419, respectively. In addition, this study provides concrete figures for the burden of obesity in Italy at a population level (Table ), while previous studies only reported an increased percentage of healthcare utilisation or average costs pro-capita [29, 90]. Finally, the study results were validated by performing a deterministic sensitivity analysis and a PSA as discussed above.
This study presents limitations that need to be considered. First, children and adolescents were excluded from the analysis due to the heterogeneity of input data sources, thus underestimating the real burden of obesity in Italy. In addition, this study does not consider variations in direct costs between obesity classes (I, II, III). Therefore, this study does not account for the fact that severely obese patients (class III) have been reported to display an increased risk for several CVDs and multi-morbidity compared to class I and II patients and therefore have a greater impact on the overall burden of obesity [91]. Most of the RRs used for the PAFs were collected in the US, and transferring these risk measures to Italy may result in uncertainty (Tables and ). Moreover, this study does not consider that obese people often present multiple comorbidities that have a synergistic rather than additive effect and display specific dynamics that might not be reflected in the PAF. Another limitation of the PAF is its consideration of all patients affected by a certain pathology on the same level regardless of the exposure (e.g. obese or not obese). However, a normal-weight patient with a single pathology costs on average less than an obese patient with the same pathology. Choosing for example a cost pro-capita of 353 solely attributable to diabetes represents a highly prudential choice because an obese patient affected by diabetes holds a higher probability to be contemporary affected by a CVD compared to a normal-weight diabetic. The use of the PAF may also lead to accounting costs of the included pathologies more than once. For example, using the topdown approach to estimate the costs of the comorbidity diabetes and retrieving the value from another study would lead to accounting also for costs attributed to CVDs resulted from diabetes, which in our study are considered separately. To minimise the risk of double counting, we filtered out cost categories referring to comorbidities when extracting the costs pro-capita for each pathology from the literature. Finally, an average cost per person for all cancers was considered for the cost evaluation due to the lack of country-specific data in the literature. However, the assumption of an equal cost per person among different cancer types might have resulted in a simplification and consequent underestimation or overestimation of costs.
For future research, it is advisable to include other pathologies (e.g. dyslipidaemia and arthritis), and consider cost figures specific for each type of obesity-associated pathology included in the analysis (i.e. specific costs for each included cancer type). It is also advisable to include children and adolescents, excluded in this study due to the lack of suitable data for Italy, at present displaying a marked heterogeneity in collection methods, as well as in the definitions of age cutoffs and BMI classes. We obtained data for this study using a snow-ball method instead of a systematic review approach due to the fact that we started our search based on three key national reports that provided specific figures for Italy. A snow-ball method approach appeared more effective since it allowed us to start from a number of key references and gradually widen the scope.
In conclusion, this study represents the first comprehensive COI study of obesity in Italy which estimated direct healthcare costs of the most common obesity-associated pathologies and indirect costs due to productivity losses. The findings indicated high obesity-attributable costs, calling for action on developing cost-effective prevention programmes in Italy. This study also underlines the lack of reliable data on obesity prevalence for Italy, which constitutes the basis for sound economic evaluations. Further effort should be put in developing more reliable data collection to improve homogeneity and comparability of results and to reach a national consensus on the cost of obesity.
References
1.
Kahn SE, Hull RL, Utzschneider KM. Mechanisms linking obesity to insulin resistance and type 2 diabetes. Nature. 2006;444(7121):840846. doi:10.1038/nature05482. [PubMed] [CrossRef] [Google Scholar]2.
Ortega FB, Lavie CJ, Blair SN. Obesity and cardiovascular disease. Circ. Res. 2016;118(11):17521770. doi:10.1161/circresaha.115.306883. [PubMed] [CrossRef] [Google Scholar]3.
Smith SC., Jr Multiple risk factors for cardiovascular disease and diabetes mellitus. Am. J. Med. 2007;120(3 Suppl 1):S3S11. doi:10.1016/j.amjmed.2007.01.002. [PubMed] [CrossRef] [Google Scholar]4.
Luppino FS, de Wit LM, Bouvy PF, Stijnen T, Cuijpers P, Penninx BW, et al. Overweight, obesity, and depression: a systematic review and meta-analysis of longitudinal studies. Arch. Gen. Psychiatry. 2010;67(3):220229. doi:10.1001/archgenpsychiatry.2010.2. [PubMed] [CrossRef] [Google Scholar]5.
Jantaratnotai N, Mosikanon K, Lee Y, McIntyre RS. The interface of depression and obesity. Obes. Res. Clin. Pract. 2017;11(1):110. doi:10.1016/j.orcp.2016.07.003. [PubMed] [CrossRef] [Google Scholar]6.
Ng M, Fleming T, Robinson M, Thomson B, Graetz N, Margono C, et al. Global, regional, and national prevalence of overweight and obesity in children and adults during 19802013: a systematic analysis for the Global Burden of Disease Study 2013. Lancet (London, England) 2014;384(9945):766781. doi:10.1016/s0140-6736(14)60460-8. [PMC free article] [PubMed] [CrossRef] [Google Scholar]8.
Meldrum DR, Morris MA, Gambone JC. Obesity pandemic: causes, consequences, and solutions-but do we have the will? Fertil. Steril. 2017;107(4):833839. doi:10.1016/j.fertnstert.2017.02.104. [PubMed] [CrossRef] [Google Scholar]9. The heavy burden of obesity: the economics of prevention. In: Publishing, O. (ed.) PECD Health Policy Studies. OECD, Paris (2019)
10.
Pineda E, Sanchez-Romero LM, Brown M, Jaccard A, Jewell J, Galea G, et al. Forecasting future trends in obesity across Europe: the value of improving surveillance. Obes. Facts. 2018;11(5):360371. doi:10.1159/000492115. [PMC free article] [PubMed] [CrossRef] [Google Scholar]11.
Dee A, Kearns K, O'Neill C, Sharp L, Staines A, O'Dwyer V, et al. The direct and indirect costs of both overweight and obesity: a systematic review. BMC Res. Notes. 2014;7:242. doi:10.1186/1756-0500-7-242. [PMC free article] [PubMed] [CrossRef] [Google Scholar]12. Obesity and the Economics of Prevention: Fit not FatItaly Key Facts. (2020). Accessed 6 July 2020
13. Atella, V., Kopinska, J.: I costi dellobesit in Italia. In: Fondazione economia Tor Vergata (2012)
14. dAngela, D., Giordani, C., Polistena, B., Spandonaro, F.: Dati Economici. In: Obesity Barometer Monitor, pp. 7986 (2019)
16. Foschi, D., Moroni, R., De Luca, M., Navarra, S., Sarro, G., Foletto, M., et al.: Linee guida di chirurgia dellobesit. In: Societ Italiana di Chirurgia dellObesit e delle malattie metaboliche (SICOB) (2016)
17. 1 Italian Obesity Barometer Report. In: Italian Barometer Diabetes Observatory (IBDO) Foundation (2019)
18.
Panico S, Palmieri L, Donfrancesco C, Vanuzzo D, Chiodini P, Cesana G, et al. Preventive potential of body mass reduction to lower cardiovascular risk: the Italian Progetto CUORE study. Prev. Med. 2008;47(1):5360. doi:10.1016/j.ypmed.2008.01.023. [PubMed] [CrossRef] [Google Scholar]19.
Pellai A, Nobile M, Luti C. A quali-quantitative analysis of food advertising in Italian Children's National TV Programming: implications for school based nutrition educational programs. J. Nutr. Therap. 2012;1:8285. [Google Scholar]20.
Bray GA, Kim KK, Wilding JPH. Obesity: a chronic relapsing progressive disease process. A position statement of the World Obesity Federation. Obesity Rev. 2017;18(7):715723. doi:10.1111/obr.12551. [PubMed] [CrossRef] [Google Scholar]21.
Upadhyay J, Farr O, Perakakis N, Ghaly W, Mantzoros C. Obesity as a disease. Med. Clin. North Am. 2018;102(1):1333. doi:10.1016/j.mcna.2017.08.004. [PubMed] [CrossRef] [Google Scholar]22.
Caterson ID, Alfadda AA, Auerbach P, Coutinho W, Cuevas A, Dicker D, et al. Gaps to bridge: misalignment between perception, reality and actions in obesity. Diabetes Obes. Metab. 2019;21(8):19141924. doi:10.1111/dom.13752. [PMC free article] [PubMed] [CrossRef] [Google Scholar]25.
Tarricone R. Cost-of-illness analysis. What room in health economics? Health Policy. 2006;77(1):5163. doi:10.1016/j.healthpol.2005.07.016. [PubMed] [CrossRef] [Google Scholar]26.
Costa N, Derumeaux H, Rapp T, Garnault V, Ferlicoq L, Gillette S, et al. Methodological considerations in cost of illness studies on Alzheimer disease. Heal. Econ. Rev. 2012;2(1):18. doi:10.1186/2191-1991-2-18. [PMC free article] [PubMed] [CrossRef] [Google Scholar]27.
Frood S, Johnston LM, Matteson CL, Finegood DT. Obesity, complexity, and the role of the health system. Curr. Obes. Rep. 2013;2(4):320326. doi:10.1007/s13679-013-0072-9. [PMC free article] [PubMed] [CrossRef] [Google Scholar]28.
Tremmel M, Gerdtham UG, Nilsson PM, Saha S. Economic burden of obesity: a systematic literature review. Int. J. Environ. Res. Public Health. 2017;14(4):435. doi:10.3390/ijerph14040435. [PMC free article] [PubMed] [CrossRef] [Google Scholar]29.
Atella V, Kopinska J, Medea G, Belotti F, Tosti V, Mortari AP, et al. Excess body weight increases the burden of age-associated chronic diseases and their associated health care expenditures. Aging. 2015;7(10):882892. doi:10.18632/aging.100833. [PMC free article] [PubMed] [CrossRef] [Google Scholar]30.
Lette M, Bemelmans WJ, Breda J, Slobbe LC, Dias J, Boshuizen HC. Health care costs attributable to overweight calculated in a standardized way for three European countries. Eur. J. Health Econ. 2016;17(1):6169. doi:10.1007/s10198-014-0655-8. [PMC free article] [PubMed] [CrossRef] [Google Scholar]31. Fattori di rischio per la salute: fumo, obesit, alcol e sedentariet. In: Italian Institute for Statistics (2016)
32.
Gold M, Siegel J, Russel L, Weinstein M. Cost-effectiveness in Health and Medicine. New York: Oxford University Press; 1996. [Google Scholar]33.
Bloom BS, Bruno DJ, Maman DY, Jayadevappa R. Usefulness of US cost-of-illness studies in healthcare decision making. Pharmacoeconomics. 2001;19(2):207213. doi:10.2165/00019053-200119020-00007. [PubMed] [CrossRef] [Google Scholar]34.
Hodgson TA. The state of the art of cost-of-illness estimates. Adv. Health Econ. Health Serv. Res. 1983;4:129164. [PubMed] [Google Scholar]35.
Poole C. A history of the population attributable fraction and related measures. Ann. Epidemiol. 2015;25(3):147154. doi:10.1016/j.annepidem.2014.11.015. [PubMed] [CrossRef] [Google Scholar]36.
Muller D, Stock S, Dintsios CM, Chernyak N, Gerber-Grote A, Gloede TD, et al. Checklist for the development and assessment of cost-of-illness studies. Gesundheitswesen (Bundesverband der Arzte des Offentlichen Gesundheitsdienstes (Germany)) 2018;80:744753. doi:10.1055/s-0042-124664. [PubMed] [CrossRef] [Google Scholar]37. Body Mass Index (BMI). cdc.gov/healthyweight/assessing/bmi/index.html. Accessed 19 May 2020
38. Nicolucci, A., Sbraccia, P., Guerra, R., Sclaera, G., Pisanti, P., Lauro, R. et al.: Il burden of disease dellobesit in Italia. In: Health Policy in Non-communicable disease. IBDO Foundation (2015)
39. Di Zio, M., Fursova, N., Geselma, T., Gieing, S., Guarnera, U., Petrauskiene, J. et al.: Methodology for data validation 1.0. In: Essnet Validat Foundation (2016)
40.
Rowland ML. Self-reported weight and height. Am. J. Clin. Nutr. 1990;52(6):11251133. doi:10.1093/ajcn/52.6.1125. [PubMed] [CrossRef] [Google Scholar]41.
Spencer EA, Appleby PN, Davey GK, Key TJ. Validity of self-reported height and weight in 4808 EPIC-Oxford participants. Public Health Nutr. 2002;5(4):561565. doi:10.1079/phn2001322. [PubMed] [CrossRef] [Google Scholar]42.
Niedhammer I, Bugel I, Bonenfant S, Goldberg M, Leclerc A. Validity of self-reported weight and height in the French GAZEL cohort. Int. J. Obes. Relat. Metab. Disorders. 2000;24(9):11111118. doi:10.1038/sj.ijo.0801375. [PubMed] [CrossRef] [Google Scholar]43. DAngela, D., Cambiano, C., Glorioso, V.: PariSanitOsservatorio per lequit di accesso alle prestazioni. In: Assobiomedica, C.R.E.A. Sanit (2020)
44.
Giampaoli S, Palmieri L, Donfrancesco C, Lo Noce C, Pilotto L, Vanuzzo D. Cardiovascular health in Italy. Ten-year surveillance of cardiovascular diseases and risk factors: Osservatorio Epidemiologico Cardiovascolare/Health Examination Survey 19982012. Eur. J. Prevent. Cardiol. 2015;22(2 Suppl):937. doi:10.1177/2047487315589011. [PubMed] [CrossRef] [Google Scholar]45.
Lauby-Secretan B, Scoccianti C, Loomis D, Grosse Y, Bianchini F, Straif K. Body fatness and cancerviewpoint of the IARC Working Group. N. Engl. J. Med. 2016;375(8):794798. doi:10.1056/NEJMsr1606602. [PMC free article] [PubMed] [CrossRef] [Google Scholar]46.
Wilson PWF, D'Agostino RB, Sullivan L, Parise H, Kannel WB. Overweight and obesity as determinants of cardiovascular risk: the Framingham experience. Arch. Intern. Med. 2002;162(16):18671872. doi:10.1001/archinte.162.16.1867. [PubMed] [CrossRef] [Google Scholar]47.
Guh DP, Zhang W, Bansback N, Amarsi Z, Birmingham CL, Anis AH. The incidence of co-morbidities related to obesity and overweight: a systematic review and meta-analysis. BMC Public Health. 2009;9:88. doi:10.1186/1471-2458-9-88. [PMC free article] [PubMed] [CrossRef] [Google Scholar]48.
Andrade C. Understanding relative risk, odds ratio, and related terms: as simple as it can get. J. Clin. Psychiatry. 2015;76(7):e857861. doi:10.4088/JCP.15f10150. [PubMed] [CrossRef] [Google Scholar]50.
Gupta S, Richard L, Forsythe A. The humanistic and economic burden associated with increasing body mass index in the EU5. Diabetes Metab Syndrome Obesity. 2015;8:327338. doi:10.2147/dmso.s83696. [PMC free article] [PubMed] [CrossRef] [Google Scholar]51.
Borisenko O, Colpan Z, Dillemans B, Funch-Jensen P, Hedenbro J, Ahmed AR. Clinical indications, utilization, and funding of bariatric surgery in Europe. Obes. Surg. 2015;25(8):14081416. doi:10.1007/s11695-014-1537-y. [PMC free article] [PubMed] [CrossRef] [Google Scholar]52.
Desogus D, Menon V, Singhal R, Oyebode O. An Examination of who is eligible and who is receiving bariatric surgery in England: secondary analysis of the health survey for England dataset. Obes. Surg. 2019;29(10):32463251. doi:10.1007/s11695-019-03977-3. [PMC free article] [PubMed] [CrossRef] [Google Scholar]53.
Colao A, Lucchese M, D'Adamo M, Savastano S, Facchiano E, Veronesi C, et al. Healthcare usage and economic impact of non-treated obesity in Italy: findings from a retrospective administrative and clinical database analysis. BMJ Open. 2017;7(2):e013899. doi:10.1136/bmjopen-2016-013899. [PMC free article] [PubMed] [CrossRef] [Google Scholar]54. Europan Health Interview Survey. In: European Statistics (EUROSTAT) (2015)
55.
Lehnert T, Sonntag D, Konnopka A, Riedel-Heller S, Knig HH. Economic costs of overweight and obesity. Best practice & research. Clin. Endocrinol. Metab. 2013;27(2):105115. doi:10.1016/j.beem.2013.01.002. [PubMed] [CrossRef] [Google Scholar]57.
Avgerinos KI, Spyrou N, Mantzoros CS, Dalamaga M. Obesity and cancer risk: emerging biological mechanisms and perspectives. Metab. Clin. Exp. 2019;92:121135. doi:10.1016/j.metabol.2018.11.001. [PubMed] [CrossRef] [Google Scholar]58.
McDonald ME, Bender DP. Endometrial cancer: obesity, genetics, and targeted agents. Obstet. Gynecol. Clin. North Am. 2019;46(1):89105. doi:10.1016/j.ogc.2018.09.006. [PubMed] [CrossRef] [Google Scholar]59.
Tumminia, A., Vinciguerra, F., Parisi, M., Graziano, M., Sciacca, L., Baratta, R. et al.: Adipose tissue, obesity and adiponectin: role in endocrine cancer risk. Int. J. Mol. Sci. 20(12):2863 (2019). 10.3390/ijms20122863 [PMC free article] [PubMed]60.
Atella, V., Piano Mortari, A., Kopinska, J., Belotti, F., Lapi, F., Cricelli, C. et al.: Trends in age-related disease burden and healthcare utilization. Aging Cell 18(1):e12861 (2019). 10.1111/acel.12861 [PMC free article] [PubMed]61.
Moretti AM, Tafuri S, Parisi D, Germinario C. Epidemiology of pulmonary embolism in Apulia from analysis of current data. Monaldi Archiv. Chest Dis. 2010;73(1):1824. doi:10.4081/monaldi.2010.309. [PubMed] [CrossRef] [Google Scholar]62. Overweight and obesity report on data collection for overweight and obesity prevalence and related relative risks. In: International Association for the Study of Obesity (2010)
63.
Wanahita N, Messerli FH, Bangalore S, Gami AS, Somers VK, Steinberg JS. Atrial fibrillation and obesityresults of a meta-analysis. Am. Heart J. 2008;155(2):310315. doi:10.1016/j.ahj.2007.10.004. [PubMed] [CrossRef] [Google Scholar]64.
Worldwide trends in body-mass index Underweight, overweight, and obesity from 1975 to 2016: a pooled analysis of 2416 population-based measurement studies in 1289 million children, adolescents, and adults. Lancet (London, England) 2017;390(10113):26272642. doi:10.1016/s0140-6736(17)32129-3. [PMC free article] [PubMed] [CrossRef] [Google Scholar]65. I numeri del cancro in Italia. In: AIOM (2014)
66.
Marcellusi A, Viti R, Mecozzi A, Mennini FS. The direct and indirect cost of diabetes in Italy: a prevalence probabilistic approach. Eur. J. Health Econ. 2016;17(2):139147. doi:10.1007/s10198-014-0660-y. [PubMed] [CrossRef] [Google Scholar]67.
Ringborg A, Nieuwlaat R, Lindgren P, Jnsson B, Fidan D, Maggioni AP, et al. Costs of atrial fibrillation in five European countries: results from the Euro Heart Survey on atrial fibrillation. Europace. 2008;10(4):403411. doi:10.1093/europace/eun048. [PubMed] [CrossRef] [Google Scholar]68.
Corrao G, Ghirardi A, Ibrahim B, Merlino L, Maggioni AP. Burden of new hospitalization for heart failure: a population-based investigation from Italy. Eur. J. Heart Fail. 2014;16(7):729736. doi:10.1002/ejhf.105. [PubMed] [CrossRef] [Google Scholar]69.
Scholze J, Alegria E, Ferri C, Langham S, Stevens W, Jeffries D, et al. Epidemiological and economic burden of metabolic syndrome and its consequences in patients with hypertension in Germany, Spain and Italy; a prevalence-based model. BMC Public Health. 2010;10(1):529. doi:10.1186/1471-2458-10-529. [PMC free article] [PubMed] [CrossRef] [Google Scholar]70.
Mantovani LG, Fornari C, Madotto F, Riva MA, Merlino L, Ferrario MM, et al. Burden of acute myocardial infarction. Int. J. Cardiol. 2011;150(1):111112. doi:10.1016/j.ijcard.2011.04.030. [PubMed] [CrossRef] [Google Scholar]71.
Gussoni G, Foglia E, Frasson S, Casartelli L, Campanini M, Bonfanti M, et al. Real-world economic burden of venous thromboembolism and antithrombotic prophylaxis in medical inpatients. Thromb. Res. 2013;131(1):1723. doi:10.1016/j.thromres.2012.10.008. [PubMed] [CrossRef] [Google Scholar]72.
Gerzeli S, Tarricone R, Zolo P, Colangelo I, Busca MR, Gandolfo C. The economic burden of stroke in Italy The EcLIPSE Study: economic longitudinal incidence-based project for stroke evaluation. Neurol. Sci. 2005;26(2):7280. doi:10.1007/s10072-005-0439-0. [PubMed] [CrossRef] [Google Scholar]73.
Jnsson B, Hofmarcher T, Lindgren P, Wilking N. The cost and burden of cancer in the European Union 19952014. Eur. J. Cancer (Oxford, England: 1990) 2016;66:162170. doi:10.1016/j.ejca.2016.06.022. [PubMed] [CrossRef] [Google Scholar]74.
Peterson MD, Mahmoudi E. Healthcare utilization associated with obesity and physical disabilities. Am. J. Prev. Med. 2015;48(4):426435. doi:10.1016/j.amepre.2014.11.007. [PMC free article] [PubMed] [CrossRef] [Google Scholar]75.
Nrtoft E, Chubb B, Borglykke A. Obesity and healthcare resource utilization: comparative results from the UK and the USA. Obes. Sci. Pract. 2017;4(1):4145. doi:10.1002/osp4.148. [PMC free article] [PubMed] [CrossRef] [Google Scholar]76.
Drummond M. Cost-of-illness studies: a major headache? Pharmacoeconomics. 1992;2(1):14. doi:10.2165/00019053-199202010-00001. [PubMed] [CrossRef] [Google Scholar]77.
Konnopka A, Bdemann M, Knig HH. Health burden and costs of obesity and overweight in Germany. Eur. J. Health Econ. 2011;12(4):345352. doi:10.1007/s10198-010-0242-6. [PubMed] [CrossRef] [Google Scholar]78.
Runkel N, Colombo-Benkmann M, Httl TP, Tigges H, Mann O, Sauerland S. Bariatric surgery. Dtsch. Arztebl. Int. 2011;108(20):341346. doi:10.3238/arztebl.2011.0341. [PMC free article] [PubMed] [CrossRef] [Google Scholar]79.
Oberlin P, de Peretti C. Bariatric surgery in France from 1997 to 2018. Surg. Obesity Relat. Dis. 2020;16(8):10691077. doi:10.1016/j.soard.2020.04.022. [PubMed] [CrossRef] [Google Scholar]80.
Goettler A, Grosse A, Sonntag D. Productivity loss due to overweight and obesity: a systematic review of indirect costs. BMJ Open. 2017 doi:10.1136/bmjopen-2016-014632. [PMC free article] [PubMed] [CrossRef] [Google Scholar]81.
Effertz T, Engel S, Verheyen F, Linder R. The costs and consequences of obesity in Germany: a new approach from a prevalence and life-cycle perspective. Eur. J. Health Econ. 2016;17(9):11411158. doi:10.1007/s10198-015-0751-4. [PubMed] [CrossRef] [Google Scholar]82. Knoll, K., Hauner, H.: A health-economic analysis of the total cost burden caused by obesity and the diseases associated with obesity in the Federal Republic of Germany. Adipositas (2008)
83. How does Germany compare? Health at a Glance: Europe. In: Organisation for Economic Co-operation and Development (OECD) (2017)
85. Cartabellotta, N., Cottafava, E., Luceri, R., Mosti, M.: Il definanziamento 20102019 del Servizio Sanitario Nazionale. In: Report Osservatorio GIMBE n. 7/2019 (2019)
86.
Klarenbach S, Padwal R, Chuck A, Jacobs P. Population-based analysis of obesity and workforce participation. Obesity (Silver Spring, MD) 2006;14(5):920927. doi:10.1038/oby.2006.106. [PubMed] [CrossRef] [Google Scholar]87.
Ricci JA, Chee E. Lost productive time associated with excess weight in the US workforce. J. Occup. Environ. Med. 2005;47(12):12271234. doi:10.1097/01.jom.0000184871.20901.c3. [PubMed] [CrossRef] [Google Scholar]88.
Kaplan HC, Brady PW, Dritz MC, Hooper DK, Linam WM, Froehle CM, et al. The influence of context on quality improvement success in health care: a systematic review of the literature. Milbank Q. 2010;88(4):500559. doi:10.1111/j.1468-0009.2010.00611.x. [PMC free article] [PubMed] [CrossRef] [Google Scholar]89.
Tomoaia-Cotisel A, Scammon DL, Waitzman NJ, Cronholm PF, Halladay JR, Driscoll DL, et al. Context matters: the experience of 14 research teams in systematically reporting contextual factors important for practice change. Ann. Fam. Med. 2013;11(Suppl 1):S115S123. doi:10.1370/afm.1549. [PMC free article] [PubMed] [CrossRef] [Google Scholar]90.
Migliore E, Pagano E, Mirabelli D, Baldi I, Gregori D, Zocchetti C, et al. Hospitalization rates and cost in severe or complicated obesity: an Italian cohort study. BMC Public Health. 2013;13:544. doi:10.1186/1471-2458-13-544. [PMC free article] [PubMed] [CrossRef] [Google Scholar]91.
Patil H, Astik G, House JA, O'Keefe JH, Main ML. Prevalence of grade II and III obesity among patients hospitalized with cardiovascular diagnoses in 2002 v 2009. Missouri Med. 2012;109(5):397401. [PMC free article] [PubMed] [Google Scholar]92. Body Mass Index (BMI). who.int/data/themes/theme-details/GHO/body-mass-index-(bmi)?introPage=intro_3.html (2016). Accessed 15 May 2020
93. Indagine multiscopo: Aspetti della vita quotidiana. In: Istituto nazionale di statistica (ISTAT) (2018)
94. Indagine Sorveglianza Passi. In: EpiCentro (2018)